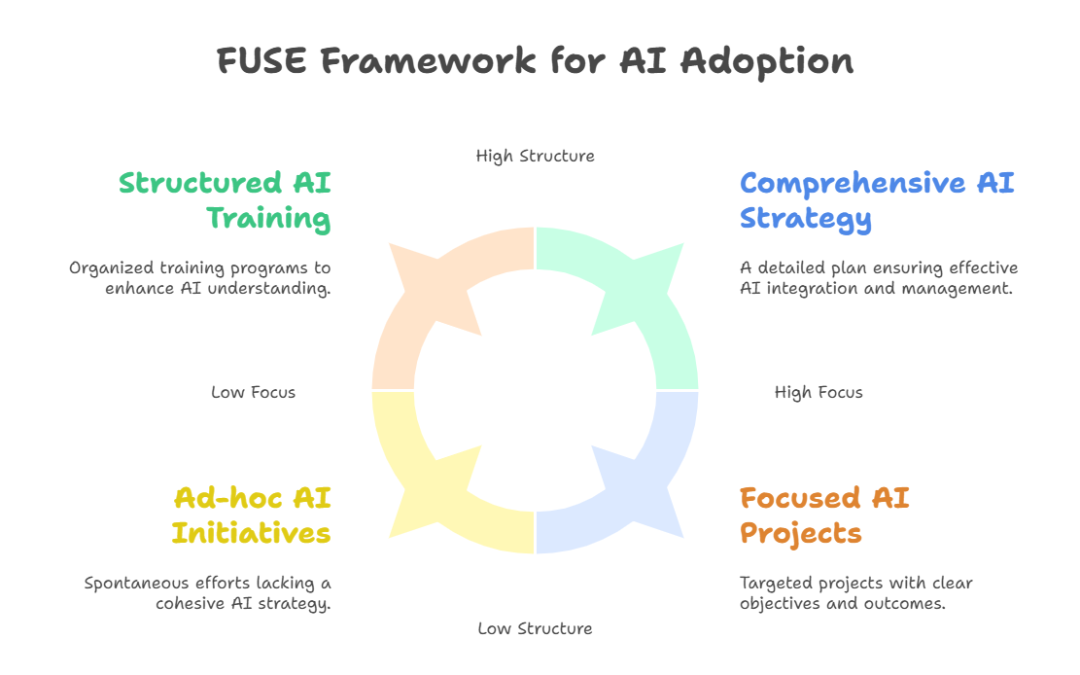
Mitigating AI Pilot Fatigue: A Structured Approach to AI Adoption with the FUSE Framework
Many organizations are finding themselves stuck in a cycle of failed projects, struggling to transition from experimentation to practical implementation. Here is what the research says on “AI Project Failure Rates:”
- Research from Gartner indicates that over 80% of AI projects fail to deliver significant business value, often due to a lack of clear strategy and alignment with business goals.
- Budget Overruns: A survey by Deloitte found that 70% of AI projects exceed their initial budget estimates, with organizations often spending 20-30% more than planned.
- Time Overruns: According to McKinsey, 60% of AI initiatives experience delays, with many taking 25-50% longer than initially projected to implement.
- Return on Investment (ROI): A PwC report highlights that only about 40% of organizations see a positive ROI from their AI investments, with many struggling to quantify the benefits.
- Data Quality Issues: A survey by O’Reilly Media found that 70% of data scientists identify poor data quality as a significant barrier to successful AI project implementation, affecting model performance.
- Integration Challenges: IBM reports that 60% of organizations face difficulties in integrating AI solutions into existing systems, which can lead to project failures or suboptimal outcomes.
- Skill Gaps: LinkedIn’s Workforce Report states that 54% of companies struggle to find talent with the necessary skills in AI and machine learning, hindering project success.
If you’re facing AI pilot fatigue, don’t worry—you’re not alone. But the key to overcoming this hurdle is adopting a structured framework designed for sustainable success. Enter the FUSE Framework: a methodical, comprehensive approach that ensures AI adoption aligns with your business goals, mitigates risks, and drives meaningful outcomes.
Tackling AI Pilot Fatigue: A More Focused Approach
The era of broad generative AI experimentation is evolving. Organizations are shifting from broad, uncoordinated initiatives to more focused, strategic investments aimed at solving business-critical challenges.
A recent NTT DATA survey found that 90% of senior decision-makers experience “pilot fatigue,” largely due to poor data readiness, immature technology, and unproductive outcomes from early-stage AI initiatives.As a result, many companies are rethinking their strategies, focusing their efforts on fewer, targeted pilots that align directly with their core business needs.
“Pilot fatigue, aimless experimentation, and failure rates have many organizations shifting generative AI investments toward more targeted — and promising — business use cases.” reports CIO
Instead of investing resources into generic AI applications like chatbots or HR tools, businesses are focusing on specific use cases that deliver clear, measurable value—such as improving productivity, reducing costs, and enhancing the customer experience. This pivot is essential for overcoming pilot fatigue and avoiding the drain on resources and morale that comes from aimless experimentation.
By narrowing their focus, businesses are ensuring that AI delivers real, lasting ROI
Strategic Investments in Generative AI: A Shift Toward High-Value Use Cases
Despite mixed early results, spending on generative AI continues to rise. In fact, 61% of organizations plan to significantly increase their investments in the next two years. The focus has shifted from broad experimentation to implementing AI governance frameworks, which help companies strategically align their investments with tangible business goals. Industry experts agree that the most successful AI initiatives arise from clear, well-defined goals—such as improving customer experience, increasing operational efficiency, or boosting revenue. By focusing on high-value, industry-specific use cases, businesses can bridge the gap between AI’s potential and its meaningful application.
Fusefy’s Approach: Turning AI Potentials into Real Results
AI has the potential to revolutionize industries by automating workflows, improving decision-making, and driving innovation. However, realizing these benefits requires overcoming several implementation challenges. Issues like limited resources, data security concerns, and a lack of transparency can all hinder AI adoption.
Fusefy addresses these barriers head-on with its AI Adoption as a Service (AIaaS) model, powered by the FUSE framework. This structured approach focuses on four essential pillars: Feasibility, Usability, Security, and Explainability.
- Feasibility: The FUSE Framework starts by evaluating your organization’s readiness for AI. It assesses your infrastructure, data readiness, and team expertise to determine whether they are capable of supporting AI’s demands. By customizing AI solutions to fit your specific business needs, FUSE ensures a smoother and more successful implementation.
- Usability: To ensure smooth integration, FUSE emphasizes designing AI tools that are user-friendly and intuitive. With a user-centric design, the technology becomes a natural extension of daily workflows. Robust training programs and ongoing support ensure employees adopt AI confidently, which is key to sustaining momentum in AI adoption.
- Security: AI systems handle sensitive data, so robust security measures are critical. FUSE prioritizes data protection through encryption and ensures compliance with industry regulations like GDPR or HIPAA. This guarantees data security while maintaining trust with stakeholders.
- Explainability: Transparency in AI decision-making builds trust. The FUSE framework emphasizes the importance of understanding how AI systems make decisions, which fosters confidence and supports ethical practices. This is especially important in sectors like hiring, healthcare, and finance, where fairness and accountability are paramount.
Unlocking the Full Potential of AI
The FUSE Framework is designed to reduce the Total Cost of Ownership (TCO) while enhancing Return on Investment (ROI) by focusing on four key pillars: Feasibility, Usability, Security, and Explainability. This framework enables organizations to minimize costs associated with technology adoption while maximizing value through a structured approach.
Additionally, Fusefy’s ROI Intelligence allows organizations to evaluate ROI across four dimensions: Cost Reduction, Resource Reduction, Time Reduction, and Revenue Increase. Key metrics for these dimensions include total cost savings, percentage resource usage reduction, labor cost savings, and additional revenue generated. Influencing factors encompass operational efficiency, automation of tasks, energy efficiency, process optimization, and customer retention strategies.
Furthermore,Fusefy’s AI Ideation Studio offers specialized consulting services through AI Design Thinking workshops that prioritize use cases, design secure architectures, create comprehensive roadmaps, and deliver targeted TCO and ROI strategies. By integrating these methodologies and tools, organizations can effectively navigate the complexities of AI adoption and ensure that their investments yield substantial business impact.
Conclusion
A structured approach ensures that AI adoption is both purposeful and aligned with your business objectives. As organizations narrow their focus on high-value AI use cases, they can overcome pilot fatigue, drive innovation, and realize the full potential of AI technology. With FUSE, businesses can transform AI from a buzzword into a tangible, impactful strategy that accelerates growth and ensures long-term success.
AUTHOR
Gowri Shanker
@gowrishanker
Gowri Shanker, the CEO of the organization, is a visionary leader with over 20 years of expertise in AI, data engineering, and machine learning, driving global innovation and AI adoption through transformative solutions.